Generative AI has emerged as a powerful force reshaping the business landscape. As we stand at the threshold of a new era in technology, the trajectory of Generative AI is nothing short of meteoric. According to Forrester’s forward-looking forecast, Generative AI is poised to experience an astonishing 36% annual growth rate until 2030, securing its position as the dominant force in the AI software market, with a remarkable 55% market share. The next five years are expected to witness an unprecedented surge in Generative AI. Gartner predicts that, by 2024, nearly 40% of enterprise applications will seamlessly incorporate conversational AI—a remarkable leap from the mere 5% adoption rate in 2020.
As enterprises race to harness the potential of GenAI, it’s crucial to understand its development principles and the architectural framework underpinning its deployment. In this blog, we delve into the world of Generative AI, exploring its transformative potential, guiding principles, and a reference architecture that will revolutionize how businesses operate.
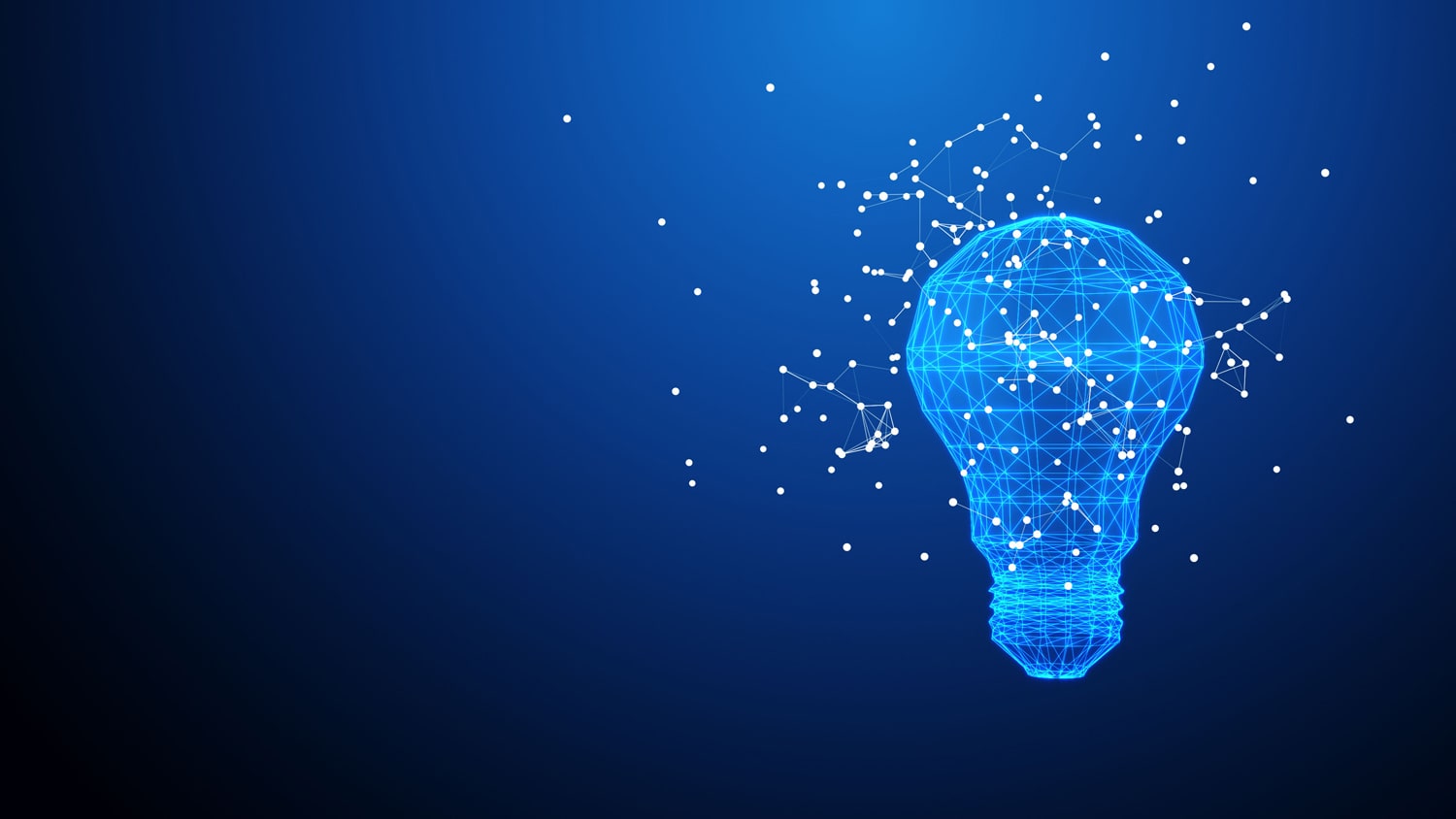
Here’s What You Will Learn in This Blog
Generative AI: A Rising Star
Generative AI has quickly become the year’s buzzword, captivating both the curiosity and apprehension of individuals and businesses alike. The technology, which harnesses cutting-edge techniques and vast data, including the prowess of large language models, can create new content across various mediums such as text, video, images, audio, and even code. It all begins with inputs, often in the form of natural language prompts or unconventional data sources, setting in motion a creative process that pushes the boundaries of AI’s achievable goals.
While some voices have raised concerns about Generative AI, its potential to augment and amplify business capabilities cannot be underestimated. Enterprises, regardless of their vertical or stage of maturity, stand at the precipice of a transformative moment. Generative AI presents many possibilities, from improving content pipelines to expediting application and data development, enhancing communication, and fostering collaboration. The time to embrace Generative AI is now—an opportunity to harness the potential of this technology and unlock new frontiers in innovation and productivity.
Generative AI Capabilities
Generative AI’s potential for enterprises is nothing short of transformative, promising a myriad of Generative AI use cases that can elevate productivity, enhance customer experiences, and unlock unprecedented innovation.
The applications of Generative AI are diverse, offering a vast and ever-expanding array of possibilities. Businesses across industries are at the forefront of this technological revolution, experimenting with innovative ways to seamlessly integrate Generative AI into their operations. The driving force behind this adoption wave is the relentless pursuit of heightened efficiency and superior decision-making capabilities, two indispensable facets in today’s fast-paced business environment. Generative AI, with its unique capabilities, not only enhances user experiences but also holds the power to streamline costs and turbocharge revenue generation, positioning it as an invaluable asset for enterprises. While we have explored some of GenAI use cases in the previous blog, it’s worth noting that Generative AI applications are an evolving frontier that continues to unveil new opportunities.
Furthermore, companies are increasingly recognizing the strategic significance of AI training as they chart their courses toward the future. Data Bricks’ findings reveal that AI Training ranks among the highest strategic priorities for companies, a trend that is expected to persist until 2027. This underscores the pivotal role that training and upskilling play in harnessing the full potential of Generative AI and other AI technologies, ensuring that businesses remain at the forefront of innovation.
As organizations delve into the realm of Generative AI, it’s paramount to adhere to a set of guiding principles that ensure responsible, ethical, and effective deployment. These principles lay the foundation for harnessing Generative AI’s capabilities while safeguarding against potential risks and pitfalls.
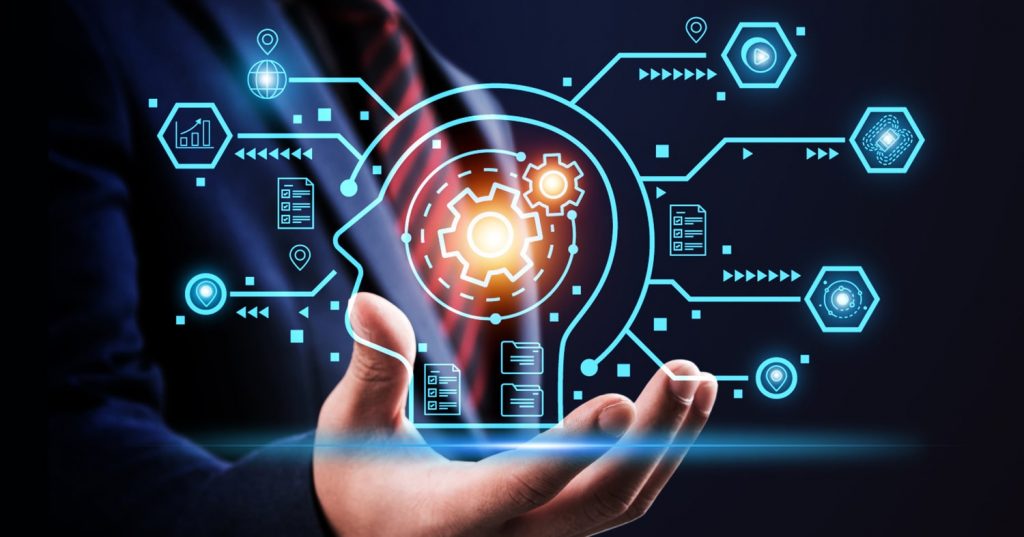
Guiding Principles for Generative AI
The guiding principles for Generative AI encompass a set of fundamental values and practices that underpin the responsible development and deployment of AI systems. Let’s discuss each of these principles and their significance:
Accountability: Accountability in GenAI refers to the practice of ensuring transparency, responsibility, and ethical governance throughout the lifecycle of artificial intelligence systems. This concept places a strong emphasis on documentation and oversight to mitigate risks and promote trust in AI technologies.
Transparency: Transparency is a cornerstone of responsible AI. AI systems should be designed to be transparent and comprehensible. This means users should be informed about the AI’s operation and decision-making processes. Transparency helps establish trust, especially when dealing with AI algorithms that rely on data. Users, influencers, and those affected by AI decisions should be able to understand how the system operates and the potential biases or risks associated with its use. By making AI transparent, we can mitigate concerns related to data-driven decisions and ensure accountability.
Fairness: AI systems must treat all individuals and demographic groups fairly. The goal is to provide consistent and equitable service to everyone, regardless of their background or characteristics. Avoiding unfair bias is crucial. Without a deliberate focus on fairness at every stage of AI system development, unintended inequalities may emerge, causing harm to individuals. Recognizing where bias can enter the AI systems and addressing it is essential to uphold the principle of fairness.
Inclusivity: AI systems should be inclusive, catering to a diverse range of users. This involves adhering to enterprise accessibility standards to ensure that the technology is effective and accessible to all, irrespective of their abilities, disabilities, or needs. Inclusivity ensures that AI benefits a broad user population, fostering a more equitable technological landscape.
Microservices: Leveraging AI microservices within the enterprise context is a strategic approach. It involves rapidly building applications and integrating AI-based software services into existing legacy systems. This approach enhances the capabilities of these systems, making them more powerful and adaptable than before, and allowing organizations to harness AI’s potential to a greater extent.
Full Life Cycle: Supporting the entire Generative AI model development life cycle is crucial. This encompasses integrated development experiences from the initial stages of model creation to deployment and maintenance. Ensuring that the AI life cycle is well-supported facilitates the creation of robust and reliable AI solutions.
Data Quality: AI relies heavily on data, making data quality management paramount. Implementing systematic data quality practices ensures that the data used for AI systems is reliable and accurate. Quality data forms the foundation of trustworthy AI outcomes.
Data Unification: Centralizing enterprise data from various sources into a unified and real-time data repository is essential. This unification streamlines data access, making it easier to leverage for AI applications, and ensures that the AI system is working with the most up-to-date information.
Multi-Format Data: Supporting a variety of data formats, including relational data, distributed file systems, key-value stores, and legacy applications, broadens the scope of AI applications and enhances their versatility.
Data Governance and Security: Data security is paramount for AI systems. Robust encryption, multi-level user access authentication, and authorization controls safeguard enterprise data, protecting it from unauthorized access or breaches.
Multi-Cloud Deployments: Enabling multi-cloud deployment allows organizations to take advantage of differentiated services across various cloud platforms. This flexibility enhances scalability and resilience in AI applications.
End-to-End Governance: Developing a comprehensive Generative AI governance framework, emphasizing ethics, integrity, security, and continuous learning, ensures that AI is developed and deployed responsibly and ethically. This approach safeguards against misuse and fosters a culture of ethical AI within organizations.
Challenges in Implementing GenAI Solutions
It is important to bear in mind that implementing Generative AI solutions in enterprises presents a host of challenges that need to be navigated for successful adoption. These challenges can be broadly categorized into data-related issues, reliability concerns, security risks, technological complexities, customization demands, skill gaps, and several other factors:
Data Curation
The foundation of Generative AI lies in data, and enterprises often grapple with identifying suitable data sources. Labeling data for algorithms, managing and governing data, setting data policies, ensuring data security, and establishing efficient data storage solutions can be daunting. Data quality and availability are crucial for the success of AI initiatives.
Dependability
Trained Generative AI models are often perceived as “black boxes” that produce results without providing insight into their decision-making processes. This lack of transparency can lead to incorrect, harmful, or unsafe results. Ensuring the reliability of AI-generated outputs is a significant challenge.
Security Concerns
Generative AI models hosted on cloud platforms can pose security risks. These models may inadvertently leak proprietary data, intellectual property (IP), personally identifiable information (PII), and even information about model interactions. Safeguarding sensitive data and ensuring the privacy and security of AI systems is paramount.
Technical Complexity
The entire AI development lifecycle is complex, from data preparation to model training and deployment. Identifying the right computing resources for model training, selecting suitable cloud platforms, and managing the intricacies of AI algorithms pose significant technological challenges.
Extensive Customization
Enterprises have diverse business needs that often require extensive customization of base foundation models. Fine-tuning and prompt engineering require substantial time and effort to align AI systems with specific business requirements.
Talent Gap
Building and maintaining Generative AI solutions necessitate expertise in machine learning, deep learning, prompt engineering, and large language models. Many enterprises need more specialized in-house resources, making initiating and sustaining AI initiatives challenging.
Beyond the challenges mentioned above, one notable concern is the potential for inaccuracies, often referred to as ‘hallucinations.’ These instances occur when a model generates an output that is neither accurate nor relevant to the original input. Hallucinations can stem from various sources, including incomplete or ambiguous information, erroneous training data, or insufficient model architecture. This intrinsic unpredictability adds complexity to Generative AI, necessitating robust strategies to ensure the accuracy and reliability of its generated content. Furthermore, these inaccuracies may inadvertently violate copyrights and legal constraints, underlining the importance of careful implementation and oversight.
Here’s how Businesses can Adopt GenAI
In order to overcome the challenges outlined above and establish a solid foundation for the successful integration of Generative AI into their operations, enterprises should carefully deliberate and prioritize certain pivotal success factors :
1. Commitment from Top Management: Generative AI initiatives require sustained commitment from top-level executives. Ensuring that leadership remains engaged and aligned with the objectives of AI adoption is crucial for long-term success. Transparent communication of the goals and potential benefits of Generative AI to all relevant stakeholders is critical. This clarity fosters understanding and buy-in from teams across the organization, making it easier to drive adoption.
2. Realistic Planning: Proper planning and setting realistic expectations are essential. Enterprises should define clear goals, timelines, and milestones, understanding that AI adoption is a journey that requires time and resources.
3. Skill Development: Addressing skill gaps is vital. Organizations can invest in training or seek external expertise to build the necessary skills for Generative AI adoption.
4. Robust Governance: Establishing robust governance practices to oversee AI initiatives, data management, and compliance is critical for mitigating risks and ensuring ethical and secure AI operations.
5. Continuous Monitoring and Evaluation: Regularly monitoring AI systems, evaluating their performance, and making necessary adjustments are essential to maintain reliability and adapt to changing business needs.
6. Alignment with Business Strategy: Generative AI strategies should be closely aligned with broader business objectives. This alignment ensures that AI initiatives directly contribute to the organization’s core mission and goals, enhancing their overall value.
7. Stakeholder Engagement: Active participation and engagement of all stakeholders, from IT teams to business units and end-users, is essential. Involving these groups in the planning, developing, and deploying Generative AI solutions can lead to more successful outcomes and greater user acceptance.
8. Culture of Responsible AI: Building a culture of responsible AI within the organization is critical. This involves promoting ethical AI practices, accountability, and a commitment to addressing the societal impacts of AI. It ensures that AI is deployed with a focus on fairness, transparency, and safety.
Step-by step plan to Implement GenAI
Businesses must adopt a methodical and strategic approach to harness the transformative potential of GenAI. So, let’s embark on a comprehensive implementation plan that encompasses the end-to-end framework for effectively leveraging GenAI within the enterprise:
Discover
In the discovery phase, businesses begin by meticulously defining the precise business challenge that the AI use case aims to address. It’s essential to be exceptionally clear about the problem to be solved. This stage also involves identifying the specific data required for the AI model to operate effectively. Additionally, businesses need to invest in data collection, cleansing, and preparation to ensure the dataset is fit for purpose. A rigorous approach to data is paramount as it forms the foundation of the entire GenAI solution.
Build
The building phase is where the technical intricacies come into play. During this stage, organizations select the most suitable AI algorithm based on the nature of the problem. This decision requires a deep understanding of AI techniques and their applications. The subsequent steps involve the actual development and thorough testing of the AI model. Rigorous testing is crucial to ensure the model’s accuracy, reliability, and resilience. Once the model has been perfected, it is deployed into the production environment, where it can start delivering value to the business.
Track
Continuous monitoring is the lifeblood of GenAI implementation. In the tracking phase, the model’s performance is observed and assessed in real-world conditions. This monitoring is vital for early detection of any issues, ensuring that the model continues to perform at peak efficiency. Problems identified during this phase are promptly addressed, and updates are made to the model as needed. Without robust tracking and monitoring, even the most advanced AI models can falter.
Assess
In the assessment phase, the focus shifts to evaluating the impact of the AI use case on overall business performance. It’s not just about solving a specific problem; it’s about understanding how AI contributes to the larger goals of the organization. This stage involves assessing the cost-effectiveness and efficiency gains brought about by the AI solution. It also includes identifying opportunities for improvement, whether through fine-tuning the model or exploring new use cases where AI can provide additional value.
Scale
As the AI model proves its value and demonstrates its positive impact on the business, it’s time to plan for scaling. Scaling encompasses defining a clear strategy for expanding the use of AI within the organization. During this phase, organizations identify potential scalability bottlenecks, make the necessary enhancements to infrastructure and processes, and establish a framework for seamless growth. Continuous monitoring and adjustment of the scaling strategy ensure that the GenAI solution can adapt to the evolving needs and demands of the business.
This comprehensive approach to GenAI implementation is a blueprint for businesses to fully embrace the potential of artificial intelligence, fostering innovation, enhancing operational efficiency, and positioning themselves for long-term success.
Drive Successful GenAI Implementation with Nsight
Nsight can offer the expertise and guidance you need at every stage of your GenAI implementation journey. Our experts can assist in defining the precise business problem that your AI use case will address and help identify the necessary data required to feed your AI model. Our team will support you in data collection and preparation, ensuring that the foundation for your GenAI solution is robust and reliable. we’ll conduct a comprehensive assessment of the AI use case’s impact on your business, highlighting opportunities for improvement and optimization. Nsight’s scalable strategies will enable you to define and address any bottlenecks in your GenAI deployment, ensuring a smooth and efficient operation.
Ready to Unlock the Full Potential of GenAI for Your Enterprise?
Frequently Asked Questions (FAQs)
A Generative AI model is an artificial intelligence system that employs machine learning techniques to generate new data based on patterns learned from existing data, such as text, images, or other content.
Generative AI models work by training on a large dataset and learning to mimic the patterns and structures in that data. They can then generate new data similar in style and content to the training data.
A good Generative AI platform should offer data management, model training capabilities, scalability, support for various data formats, and security features to protect sensitive data.
A good Generative AI platform should offer data management, model training capabilities, scalability, support for various data formats, and security features to protect sensitive data.
The choice of Generative AI type depends on your project’s specific requirements. Text-based models are suitable for content generation, while image-based models excel in visual creativity. Select the type that aligns with your goals.
Yes, Generative AI models specialize in generating art, images, and visual content, such as DeepDream and DALL·E.
About the Author
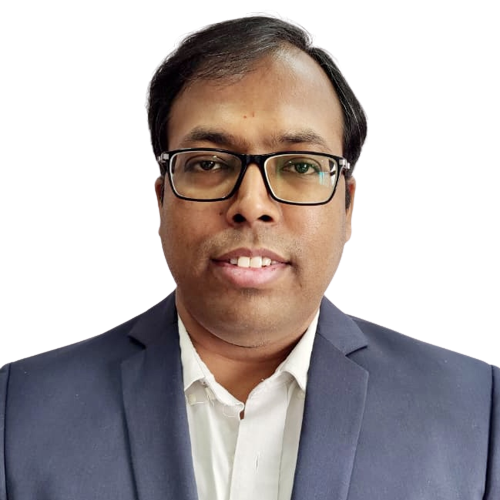
Deepak Agarwal, a digital and AI transformation expert with over 16 years of experience, is dedicated to assisting clients from various industries in realizing their business goals through digital innovation. He has a deep understanding of the unique challenges and opportunities, and he is passionate about using cutting-edge technologies to solve real-world business problems. He has a proven track record of success in helping clients improve operations, increase efficiency, and reduce costs through emerging technologies.