Studies show that early detection of anomalies can reduce operational downtime by up to 50% and save businesses millions annually in lost revenue and repair costs. Whether it’s preventing fraud—estimated to cost businesses over $5 trillion globally each year—or detecting critical system failures, the ability to identify anomalies early is essential for safeguarding time, resources, and reputation. Anomaly detection and root cause analysis are vital for maintaining the efficiency and reliability of modern systems. With advancements in artificial intelligence (AI), anomaly detection has become significantly faster, more accurate, and capable of processing vast and complex datasets in real time, ensuring businesses remain resilient and competitive.
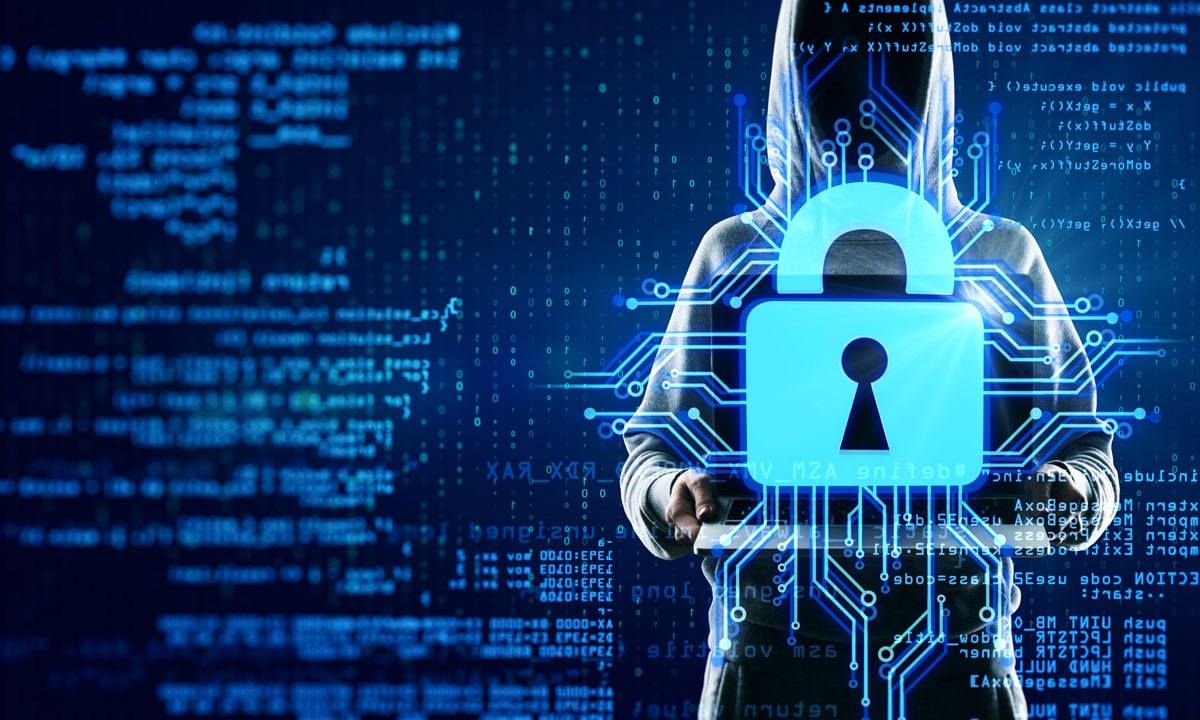
Here’s What You Will Learn in This Blog
- Overview of Anomaly Detection
- Importance of Anomaly Detection in Business
- The Transformation of Anomaly Detection Through AI
- What is Anomaly Detection?
- Examples of Anomalies Across Industries
- The Role of AI in Anomaly Detection
- Benefits of AI in Anomaly Detection
- Challenges and Considerations
- Future Trends in AI-Based Anomaly Detection
- Conclusion
Overview of Anomaly Detection
Anomaly detection refers to identifying patterns in data that deviate from the expected norm. These anomalies can signify critical issues such as system malfunctions, fraud, or security breaches. However, anomalies can also reveal opportunities, such as emerging market trends or innovative ideas hidden in the data.
Importance of Anomaly Detection in Business
Anomaly detection plays a vital role in ensuring the stability and efficiency of business operations across industries. By identifying irregularities in data, businesses can proactively address potential risks before they escalate into costly problems. Furthermore, anomaly detection systems empower businesses to make data-driven decisions. Organizations can identify operational inefficiencies by analyzing irregularities, uncover hidden opportunities, and gain insights that enhance strategic planning. For example, anomalies in customer behavior might reveal emerging market trends, enabling companies to adapt quickly and stay ahead of competitors.
The Transformation of Anomaly Detection Through AI
Artificial intelligence (AI) has fundamentally transformed anomaly detection by delivering faster, more accurate, and automated insights. Traditional anomaly detection methods, which often rely on manual processes or basic statistical models, need help with modern datasets’ growing volume and complexity. In contrast, AI-powered systems leverage advanced algorithms to process vast amounts of data in real-time, enabling businesses to identify and respond to anomalies within seconds.
AI’s ability to detect subtle patterns and correlations in data is unparalleled. For instance, deep learning models can analyze sensor data in industrial environments to predict equipment failures with up to 95% accuracy, reducing operational disruptions. AI-based systems are also adept at identifying hidden anomalies in cybersecurity, such as unusual network traffic patterns that might indicate a potential cyberattack, which can reduce breach detection time from an industry average of 207 days to just a few hours.
Additionally, AI’s automation capabilities eliminate the need for constant human monitoring, allowing businesses to allocate resources more effectively. By integrating AI into anomaly detection workflows, companies improve their operational efficiency and enhance their ability to adapt to dynamic challenges and opportunities.
What is Anomaly Detection?
Definition and Types of Anomalies
Anomaly detection involves identifying data points or patterns that deviate significantly from expected behavior. Common types of anomalies include:
Point Anomalies
Single data points differ drastically from the rest, such as a spike in temperature in a sensor reading.
Contextual Anomalies
Data that appears normal in one context but unusual in another, such as an unusual seasonal sales pattern.
Collective Anomalies
Groups of data points that, when considered together, form an anomaly, such as irregular network activity indicative of a cyberattack.
Examples of Anomalies Across Industries
Finance
In finance, AI anomaly detection systems are instrumental in identifying fraudulent transactions. These systems can detect suspicious activity in real time by analyzing deviations in spending patterns. For instance, a sudden large purchase from an unusual location or a sharp increase in account activity can trigger fraud alerts.
A 2021 report by Juniper Research estimated that AI-powered fraud detection systems could save businesses over $12 billion annually by preventing fraudulent activities.(source)
Healthcare
In healthcare, detecting rare diseases or unusual patient behavior using AI anomaly detection models has proven life-saving. AI systems can analyze vast datasets from electronic health records and wearable devices to identify anomalies that may signal undiagnosed conditions. For example, an AI model might detect irregular heart rhythms in wearable data, allowing early intervention for conditions like atrial fibrillation.
The global AI market in healthcare is expected to grow to $194.4 billion by 2030, reflecting the increasing adoption of such advanced systems.(source)
Manufacturing
Manufacturing industries benefit from AI-powered anomaly detection systems in predictive maintenance. By continuously monitoring sensor data, these systems can identify early signs of equipment failure, such as temperature spikes or unusual vibrations. For example, a smart factory may use AI to detect wear and tear in machinery, preventing costly unplanned outages.
According to McKinsey, predictive AI maintenance can reduce downtime by up to 50% and lower maintenance costs by 10-40%.(source)
IT and Security
In IT and cybersecurity, anomaly intrusion detection systems are vital for monitoring network traffic and identifying potential cyber threats. These systems can detect unusual patterns, such as unexpected login attempts or irregular data transfers, which may indicate a cyberattack. For instance, anomaly detection systems have been key in identifying ransomware attacks, preventing billions in potential losses annually.
The Ponemon Institute’s 2023 study revealed that businesses with AI-driven cybersecurity systems reduced the average data breach cost by 27%, showcasing the effectiveness of these solutions.(source)
Through these diverse applications, AI anomaly detection proves its versatility and critical importance across multiple industries, safeguarding assets, improving efficiency, and saving lives.
The Role of AI in Anomaly Detection
AI’s Improvements Over Traditional Methods
AI has elevated anomaly detection by:
● Enhancing Speed and Accuracy: AI algorithms analyze data in real time, ensuring faster anomaly detection and response.
● Automating Analysis: Automation reduces human error and provides actionable insights with minimal intervention.
AI Techniques Used in Anomaly Detection
● Machine Learning (ML): AI models leverage supervised and unsupervised learning to identify deviations in data.
● Deep Learning: Neural networks improve anomaly detection by learning from vast datasets, identifying even the most subtle anomalies.
● Generative AI: Advanced models like Generative Adversarial Networks (GANs) simulate data to detect potential anomalies effectively.
Examples of AI in Action
● Predictive Maintenance: AI systems detect anomalies in sensor data to predict equipment failures, reducing downtime and costs. Studies show that predictive maintenance can reduce maintenance costs by up to 30%.
● Fraud Detection: AI anomaly detection models spot unusual financial transactions in real time, helping banks and e-commerce platforms secure billions of dollars annually.
Benefits of AI in Anomaly Detection
Reduced Downtime and Faster Response
AI-powered anomaly detection systems drastically reduce downtime by swiftly identifying and addressing potential issues. For example, an anomaly intrusion detection system can spot and isolate a cyberattack within seconds, preventing data breaches and operational disruptions. In manufacturing, AI-based predictive maintenance systems can reduce equipment downtime by up to 50%, translating to significant productivity gains and cost savings.
Enhanced Accuracy and Decision-Making
AI systems excel at analyzing extensive datasets to detect anomalies with unparalleled precision. This capability eliminates false positives and ensures that critical issues are addressed promptly. AI anomaly detection models improve detection accuracy by up to 30% compared to traditional methods, empowering decision-makers with actionable insights. For instance, in financial fraud detection, AI achieves accuracy rates as high as 95%, enabling businesses to act confidently on identified irregularities.
Cost Savings and Operational Efficiency
Automating anomaly detection reduces reliance on manual labor, streamlines operations, and optimizes resource allocation. By automating repetitive tasks, businesses save on labor costs and allocate their human resources to higher-value activities, driving overall efficiency.
Handling Large Datasets and Complex Patterns
Modern industries generate vast amounts of data, often too complex for traditional analysis methods. AI anomaly detection models are designed to handle these massive datasets, identifying intricate patterns that may go unnoticed by human analysts. For example, in the finance sector, AI systems process millions of transactions daily, detecting fraudulent activities in real time. Similarly, in healthcare, AI models analyze patient data from wearables and medical devices to identify subtle anomalies that could indicate early signs of disease. This ability to handle complexity and scale makes AI indispensable in today’s data-driven world.
Through these benefits, AI anomaly detection is reshaping how businesses manage risks, optimize processes, and achieve long-term sustainability.
Challenges and Considerations
-
Data Quality and Availability
High-quality data is critical for the accuracy of AI anomaly detection systems. Consistent or incomplete data can compromise results and reduce system effectiveness.
-
Model Accuracy and Interpretability
AI models must balance performance and interpretability. While advanced models are highly accurate, businesses must understand the reasoning behind detected anomalies.
-
Integration with Existing Systems and Workflows
Implementing AI anomaly detection into legacy systems can be complex, requiring investments in infrastructure and training.
-
Need for Human Oversight in Critical Applications
Despite AI’s capabilities, human oversight remains essential in high-stakes applications, such as healthcare and finance, to ensure ethical and accurate decision-making.
Future Trends in AI-Based Anomaly Detection
Advancements in Explainable AI (XAI)
As AI models become more complex, the need for interpretability in anomaly detection systems grows. Explainable AI (XAI) addresses this challenge by making AI-driven anomaly detection processes more transparent and understandable. XAI techniques, such as SHAP (SHapley Additive exPlanations) and LIME (Local Interpretable Model-agnostic Explanations), help businesses understand the rationale behind anomaly detection decisions. For example, these methods can highlight which features in a dataset contributed to the classification of an anomaly, enabling domain experts to validate results and take appropriate action. This level of transparency is critical in high-stakes industries such as healthcare and finance, where decisions must be justified for compliance and ethical considerations.
Proactive Anomaly Detection with Generative AI
Generative AI transforms anomaly detection from a reactive process to a proactive one. By using models like Generative Adversarial Networks (GANs) and Variational Autoencoders (VAEs), businesses can simulate potential scenarios and predict anomalies before they occur. These models generate synthetic data that mimics real-world conditions, helping to identify vulnerabilities and potential failures in advance. For example, in cybersecurity, generative AI can simulate attack vectors to preemptively identify weak points in a network, while in manufacturing, it can anticipate equipment failures by modeling abnormal operational patterns.
This proactive approach reduces risks, minimizes downtime, and gives organizations a competitive edge by enabling them to address issues before they escalate. As generative AI continues to evolve, its integration into anomaly detection systems will lead to more predictive and adaptive solutions, further advancing the field.
Conclusion
AI-driven anomaly detection has revolutionized how businesses identify and resolve anomalies, making operations more efficient and decision-making more precise. With its ability to analyze massive datasets, detect subtle patterns, and provide actionable insights, AI systems have become indispensable tools across finance, healthcare, manufacturing, and IT industries. As artificial intelligence anomaly detection advances, its potential to streamline operations, enhance predictive capabilities, and drive innovation will only expand.
Embracing these technologies helps mitigate risks and uncovers new opportunities, ensuring businesses stay competitive in a dynamic market. Nsight into anomaly detection allows organizations to act proactively, harnessing AI’s full potential to address challenges and seize growth opportunities.
Are you ready to explore how AI anomaly detection can revolutionize your operations?
About the Author
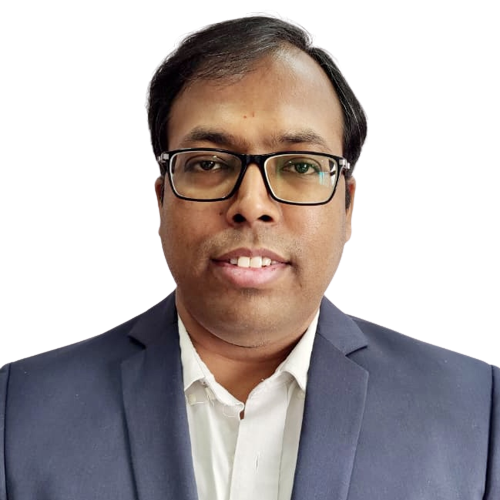
Deepak Agarwal, a digital and AI transformation expert with over 16 years of experience, is dedicated to assisting clients from various industries in realizing their business goals through digital innovation. He has a deep understanding of the unique challenges and opportunities, and he is passionate about using cutting-edge technologies to solve real-world business problems. He has a proven track record of success in helping clients improve operations, increase efficiency, and reduce costs through emerging technologies.